AI-Driven Intent Classification and Identification: Deciphering the Unspoken in User Communications
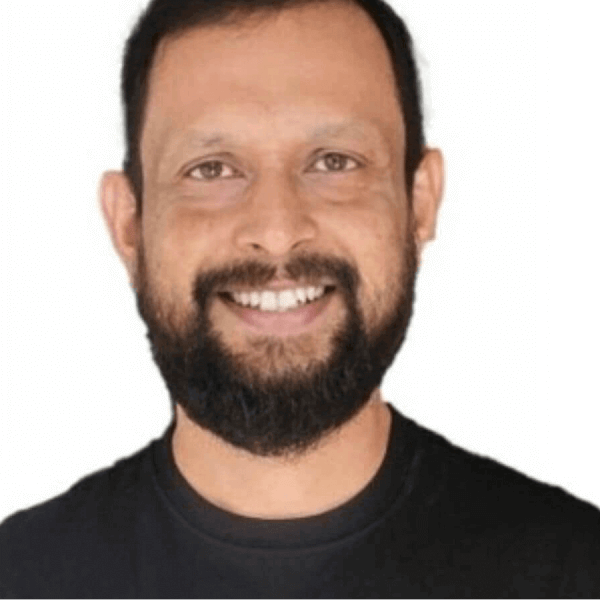
Rajesh Bhattacharjee
November 27, 2023
7 min
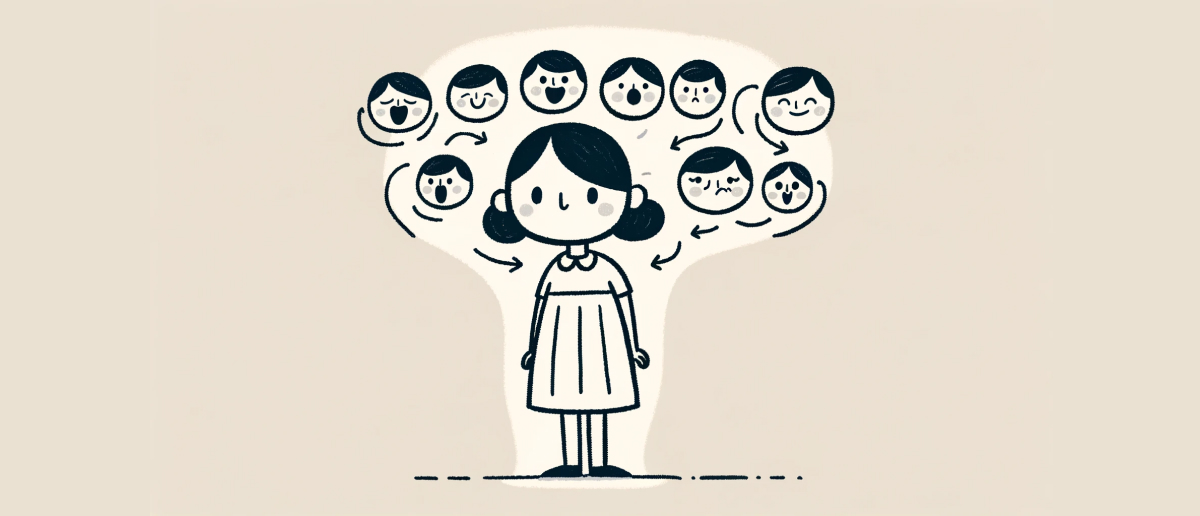
Table of Contents
The Intersection of AI and Natural Language Processing (NLP) Welcome to the fascinating world where Artificial Intelligence meets Natural Language Processing. Here, AI isn’t just about algorithms and data; it’s about understanding the nuances of human language. This blog embarks on a journey into AI-driven intent classification and identification, a cornerstone of modern NLP that allows machines to understand and react to human language meaningfully.
The Pivotal Role of Intent Classification and Identification
Intent classification is like the mind-reading skill of AI in NLP. When users interact with an AI system, they convey more than just words; they express intentions. Understanding these intentions is crucial for any AI system, like chatbots or virtual assistants, to provide relevant and accurate responses. This is where intent classification and identification come into play, bridging human input and machine interpretation.
The objective of This Exploration
We aim to dive deep into how AI powers the process of intent classification and identification. We’ll explore its mechanisms, its importance in AI-driven applications, and how it’s shaping the future of human-machine interactions. By the end of this blog, you’ll have a comprehensive understanding of this technology and its profound impact on the AI landscape.
Understanding Intent Classification and Identification
Defining Intent in the Context of AI and NLP
At its core, intent classification is how an AI system determines what a user intends or wants to achieve through their input, be it text or speech. It’s a specialized area within NLP that focuses on deciphering the purpose behind a user’s words.
A Unique Component of NLP
Intent classification differs from other NLP tasks, such as sentiment analysis, which interprets emotions, or entity recognition, which identifies key elements in the text. Instead, intent classification zeroes in on the user’s goal. For example, when someone asks a virtual assistant, “What’s the weather like today?” the intent classification system identifies the intent as seeking weather information.
The Mechanics of Intent Classification Using AI
AI Algorithms: The Engine Behind Intent Recognition Intent classification primarily employs machine learning and deep learning models. These algorithms are trained on large datasets to recognize patterns in language that signify different intents.
Processing User Input to Unveil Intent
The journey from raw user input to intent identification is intricate. Initially, the input undergoes preprocessing, including tokenization (breaking down the text into smaller parts) and stemming (reducing words to their base form). Next, the processed input is fed into an AI model. Having learned from vast examples, this model predicts the most likely intent behind the input.
Showcasing AI Models and Tools in Action
Models like LSTM (Long Short-Term Memory) networks, which are adept at processing sequential data, and BERT (Bidirectional Encoder Representations from Transformers), known for understanding the context in language, are commonly used for intent classification. These tools are chosen for their ability to grasp complex language patterns and accurately predict user intent.
Applications of Intent Classification in Various Industries
Transforming Customer Service with AI In the customer service sector, AI-driven intent classification revolutionizes how companies interact with their clients. custom ai Chatbots with intent recognition capabilities can better understand and respond to customer queries, leading to enhanced customer satisfaction and reduced response times.
Revolutionizing E-Commerce with Personalized Recommendations
E-commerce platforms leverage intent classification to understand customer needs and preferences. These platforms can offer tailored product recommendations by analyzing search queries and purchase history, enhancing the shopping experience and boosting sales.
Voice Assistants: Understanding Needs in Smart Homes
Voice assistants in smart home devices use intent classification to comprehend and execute user commands accurately. This technology enables devices to perform tasks like playing music, setting alarms, or controlling other smart devices based on user intent.
Advancing Healthcare with Patient Inquiry Analysis\
In healthcare, AI-driven intent classification helps analyze patient inquiries, thereby assisting healthcare providers in offering better patient care and streamlining administrative tasks.
Challenges in AI-based Intent Classification
Dealing with Ambiguity and Vagueness One of the primary challenges in intent classification is handling ambiguous or vague inputs where the user’s intent is unclear. AI systems must be sophisticated enough to ask follow-up questions or use context to clarify such situations.
Multilingual Content and Cultural Nuances
Another significant challenge is dealing with multilingual content and cultural nuances in language. Developing AI systems that can accurately classify intent in various languages and dialects requires extensive and diverse datasets.
Privacy and Ethical Concerns
Privacy concerns are paramount when dealing with user data, ensuring that AI systems respect user privacy and comply with data protection regulations. Additionally, ethical considerations such as transparency in AI decision-making processes are essential.
Addressing AI Biases
AI systems can inherit biases in their training data, leading to inaccurate or unfair outcomes. Regularly auditing and updating AI models to minimize biases is essential for reliable intent classification.
Improving Accuracy in Intent Classification
Enhancing AI Model Performance
Refining AI models with comprehensive and diverse training datasets is vital to improve the accuracy of intent classification. This includes incorporating various speech patterns, dialects, and colloquialisms.
The Significance of High-Quality Training Data
The effectiveness and variety within the training dataset have a direct influence on how well AI models function. It’s essential that the data accurately reflects the various situations the AI is likely to face in real-life applications.
Adopting Continuous Learning and Updating Strategies
AI models should not remain static; they must evolve with changing language use and user behaviors. Implementing continuous learning algorithms allows AI systems to adapt and improve over time.
Future Trends in Intent Classification and AI
Emerging Advancements in AI Algorithms
The future of AI in intent classification is poised to be shaped by advancements in machine learning and deep learning. Innovations like transfer learning and neural network architectures promise more accurate and efficient intent recognition.
Integrating Emotional Intelligence
A significant trend on the horizon is integrating emotional intelligence with intent classification. AI systems that can understand the intent and emotional context of user inputs will offer a more empathetic and personalized interaction.
Cross-Disciplinary AI Applications
The future will see cross-disciplinary applications of AI in intent classification. Combining intent recognition with augmented reality (AR) and virtual reality (VR) could revolutionize user experiences in gaming, education, and interactive media.
Predictive AI for Proactive Responses
Advances in predictive analytics will enable AI systems to anticipate user needs and intents, paving the way for more proactive and anticipatory user interactions. This could lead to AI systems that are reactive and predictive in their responses.
Case Studies and Real-World Examples
Chatbots in Banking
A case study of a leading bank deploying an AI-driven chatbot for customer service showcases how intent classification can streamline query resolution and enhance customer satisfaction.
AI in Retail Customer Service
An analysis of a retail giant using AI for customer inquiries demonstrates the role of intent classification in providing personalized shopping advice and support, thereby increasing customer engagement and loyalty.
Healthcare Virtual Assistants
A study of a healthcare provider using AI virtual assistants illustrates how intent classification assists in accurately triaging patient concerns, improving patient care, and reducing administrative burdens.
Conclusion
Recap of AI’s Role in Intent Classification
AI-driven intent classification and identification have emerged as pivotal technologies in understanding and responding to user needs. This journey through the intricacies of AI in intent classification has highlighted its importance, applications, challenges, and the promising future ahead.
Final Thoughts on AI Evolution
The evolution of AI in intent classification is a testament to the ongoing advancements in technology and its growing impact on every aspect of our lives. From enhancing customer experiences to streamlining operations across various industries, AI’s ability to interpret and act on user intent reshapes our interaction with technology.
Call to Action
Encouraging Exploration and Adoption
We recommend that our audience delve into the possibilities offered by AI for categorizing intentions within their respective industries. The opportunities are extensive, from boosting customer support, crafting more captivating user interactions, to augmenting process effectiveness.
Invitation for Engagement
We invite feedback, questions, or comments on this topic. Sharing experiences, insights, or inquiries about AI-driven intent classification can foster a learning and innovation community.
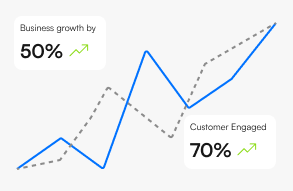
Grow Your Business with AI Chatbots
- Automate tasks
- Engage customers 24/7
- Boost conversions