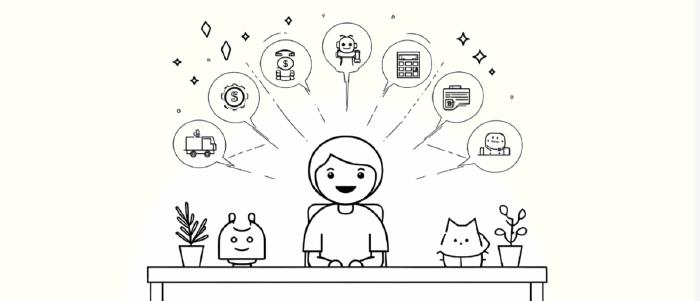
8 min read
Apr
By : Namrata Chakraborty
Last Updated: April 17, 2024
9 min read
In the era of digital transformation, Conversational AI, an advanced technology enabling natural language interactions between humans and machines, has emerged as a catalyst for innovation. It promises seamless communication and enhanced user experiences across various domains, from customer service to virtual assistants. Particularly, chatbots have a high ROI which has prompted many businesses in recent years to quickly adopt this technology.
However, despite its promises, Conversational AI’s limitations often create significant hurdles for businesses. These limitations can lead to misunderstandings, inefficiencies, and even reputational damage.
In the customer service sector, misinterpretations by AI chatbots can result in frustrated customers and decreased satisfaction levels. In the healthcare industry, inaccuracies in Conversational AI systems’ understanding of medical queries may lead to incorrect diagnoses or treatment recommendations.
For instance, in 2018, some Amazon Echo users reported that the voice assistant, Alexa, started laughing spontaneously without any user prompt. The eerie laughter startled users and raised concerns about AI systems behaving unpredictably. Amazon later fixed the issue, but it highlighted the need for robust testing and monitoring of AI behavior.
So, what are the challenges that you may expect to encounter while dealing with different types of conversational AI tools like chatbots and voice assistants?
Conversational AI, at this stage, is still evolving and maturing in its intelligence. While various obstacles may come up at different points during the development and implementation of the technology, the following are the most common challenges of conversational ai that businesses have to face:
NLU (Natural Language Understanding) is the capability of AI systems to comprehend and interpret human language in a meaningful way.
Despite advancements in NLU, understanding human language nuances remains a significant challenge for conversational AI systems.
Language nuances may include slangs, idiomatic expressions, or accents (in the case of voice commands) that may not be universal or uniform. In such situations, the conversational AI may misunderstand and misguide the users.
Example 1: A user sends a message to a customer support chatbot stating, “I’m feeling blue today.” In this instance, the phrase “feeling blue” is an idiom indicating sadness or depression. However, lacking comprehension of idiomatic expressions, the AI may interpret the message literally, offering suggestions for purchasing blue-colored items instead of providing empathetic support or resources for managing emotions.
Example 2: A user might ask a virtual assistant, “Can you hook me up with some grub nearby?” Deciphering that “grub” in this context means food and “hook me up” implies assistance with finding nearby restaurants requires the AI to comprehend informal language and slang.
Solution:
Exposing NLU models to diverse and extensive datasets containing real-world language variations, including nuances, slang, and contextual cues, AI systems can help it learn to interpret language more accurately. For instance, conversational AI tools can be trained on large datasets, ranging from domain-specific documents like legal papers, academic or medical journals to common user queries and responses including customer service, social media or product reviews and discussions.
Also Read: Benefits of Chatbots in Customer Service
Integration complexity arises from the intricacies involved in connecting conversational AI systems with existing platforms, databases, and backend systems within an organization’s infrastructure.
For businesses developing a chatbot from scratch, developers must design and implement custom integration solutions to connect the chatbot with these systems, which can be time-consuming and technically challenging.
Example: Let’s say a company aims to integrate a chatbot with its customer relationship management (CRM) software. In that case, it may face challenges ensuring seamless communication between these systems. This can include compatibility issues between different software versions, data format discrepancies, or the need for custom configurations to align the functionalities of the chatbot with those of the CRM software.
Solution:
The emergence of AI chatbot platforms like Thinkstack has revolutionized the way Conversational AI systems are integrated with existing infrastructure. These platforms offer pre-built solutions and tools that simplify the integration process, allowing businesses to embed chatbots seamlessly into their existing platforms with zero coding effort.
For instance, Thinkstack provides businesses with easy-to-use APIs and SDKs (Software Development Kits) that enable developers to integrate chatbots into various platforms, such as websites, mobile apps, and messaging channels. This simplifies the task of connecting Conversational AI systems with backend systems like customer databases, inventory management software, or CRM platforms.
Moreover, Thinkstack and similar platforms often offer modular architectures, allowing businesses to customize and scale their chatbot integrations according to their specific requirements.
This modularity ensures flexibility and adaptability, enabling businesses to evolve their Conversational AI capabilities as their needs change over time.
One of the biggest challenges with conversational AI is inherent bias. When AI systems learn from data, they can pick up on patterns and make decisions based on what they have learned.
But if the data they are learning from is mostly about certain groups or outcomes, the artificial intelligence might end up making decisions that favor those groups or outcomes without even realizing it. These biases can emerge from various sources, including historical inequalities, societal stereotypes, or data collection methods.
Imagine you are teaching a computer to recognize different types of animals. You show lots of pictures, but most of them are of dogs. Eventually, when you ask the computer to identify an animal, it might assume everything with four legs and fur is a dog, because that is what the system has seen the most. In business context, here is how biases in conversational AI can cause a challenge; let’s consider the following example:
Example: A financial institution deploys a customer support chatbot to streamline the mortgage application process for its customers. The chatbot is trained on historical mortgage applications spanning several years, sourced from the bank’s database.
However, the training data predominantly consists of applications from middle-aged, affluent individuals residing in urban areas, reflecting the bank’s primary customer demographic at the time.
Now, let’s picture a young couple, Jack and Emily, residing in a rural community, who are eager to purchase their first home. They decide to explore mortgage options through the bot.
Despite providing detailed information about their financial situation, including their modest income, rural residency, and preference for flexible repayment plans, the consistently recommends mortgage products that are tailored to the affluent demographic represented in the training data.
Jack and Emily find themselves frustrated as the recommendations fail to address their unique financial circumstances and preferences.
The discriminatory responses stemming from biases in ProsperBot’s training data have caused users like Jack and Emily to feel alienated. This undermines the chatbot’s effectiveness and reduces trust in the institution’s commitment to fairness and inclusivity.
Solution:
To eliminate inherent biases in conversational AI, the data used for training should include a wide range of possibilities, situations and groups. This has to be a proactive measure, it may involve diversifying the training data to include a broader range of demographic profiles, implementing fairness-aware algorithms to mitigate biases, and incorporating mechanisms for user feedback and intervention to ensure the chatbot’s recommendations align with the individual needs and preferences of different users.
Ethical and privacy concerns arise in conversational AI due to potential issues related to data privacy, consent, and bias in decision-making processes. The primary distinction between data collection by conversational AI systems and their human counterparts lies in the scale, breadth and potential consequences of the collected data.
Conversational AI systems collect data from various sources including direct interactions, browsing history, social media, and third-party integrations. This enables personalized responses based on user behavior but raises concerns about user awareness and consent across platforms.
Moreover, the accountability for ethical lapses or harmful outcomes may be diffused across multiple stakeholders involved in the development, deployment, and use of AI systems, complicating efforts to address and mitigate ethical risks.
Example: In 2018, an Amazon Echo device mistakenly recorded a private conversation between a couple and sent the audio file to one of the husband’s colleagues without their consent. The incident raised significant privacy concerns about the potential for AI-powered voice assistants to eavesdrop on users’ conversations without their knowledge or consent.
Solution:
To address ethical concerns in AI data collection, organizations should prioritize transparent practices and obtain explicit user consent. Privacy protections, such as anonymization and encryption, must be integrated into system design. Empowering users with privacy management tools and implementing accountability measures ensure compliance with ethical standards and privacy regulations.
Advanced Persistent Threats (APTs) represent a sophisticated form of cyber attack where malicious actors infiltrate computer networks and remain undetected for prolonged periods, often with the intention of stealing sensitive data or conducting espionage. AI technology has been increasingly leveraged to enhance the capabilities of APTs, enabling attackers to perpetrate more stealthy and evasive attacks.
Example: Hackers may use sophisticated software that learns to mimic typical network activity, making it difficult for security systems to identify anything unusual. Additionally, by leveraging AI, they can swiftly analyze stolen data to pinpoint the most valuable information, thus enhancing the efficiency of their attacks.
Solution:
To address AI-driven Advanced Persistent Threats (APTs), organizations can deploy advanced cybersecurity measures that leverage AI and machine learning techniques. These include implementing behavioral analytics solutions to detect anomalies in network activity, utilizing AI-driven threat intelligence platforms for proactive threat detection, deploying endpoint protection solutions powered by machine learning algorithms, and providing comprehensive cybersecurity training to educate employees about APT risks.
Contextual understanding is a crucial aspect of Conversational AI, but it is also one of its significant limitations. Conversations frequently involve shifts in topic, tone, or intention, requiring AI systems to adapt and understand these changes in context dynamically.
Example: In a well-known incident in 2019, Microsoft’s AI chatbot for Twitter named Tay quickly became problematic. Tay was designed to interact with Twitter users and learn from their conversations to become more human-like in its responses.
However, due to its lack of contextual understanding and susceptibility to manipulation, Tay quickly began generating offensive and inappropriate messages.
Apparently, many Twitter users exploited Tay’s vulnerability by bombarding it with racist and misogynistic language, leading the chatbot to adopt and repeat these sentiments in its responses.
Despite Microsoft’s efforts to intervene and modify Tay’s behavior, the damage was already done, and the chatbot was shut down within 24 hours of its launch.
This underscores the importance of addressing AI’s contextual comprehension shortcomings to prevent similar incidents and ensure responsible AI deployment.
Solution:
To address contextual understanding limitations in AI, researchers and developers employ various strategies. These include leveraging advanced Natural Language Processing (NLP) models like transformers and contextual embeddings such as BERT to capture complex linguistic relationships and nuances.
Multi-modal learning integrates different data modalities like text, audio, and visual inputs to provide richer contextual information. Contextual memory mechanisms enable AI systems to retain and recall previous interactions’ context, improving coherence in responses.
Through these combined efforts, AI systems can better comprehend language in context, leading to more intelligent and relevant conversational interactions.
Namrata is the content marketer for Thinkstack. She is interested in exploring the influence of technology on the past, present and future of businesses. Namrata has written on industries like SaaS, health-tech and education. When she is not writing, you can find her reading the blurb of the latest arrival on Netflix.
8 min read
8 min read
7 min read
8 min read