8 Types of AI Agents (Comparison Guide + No-Code AI Solution)
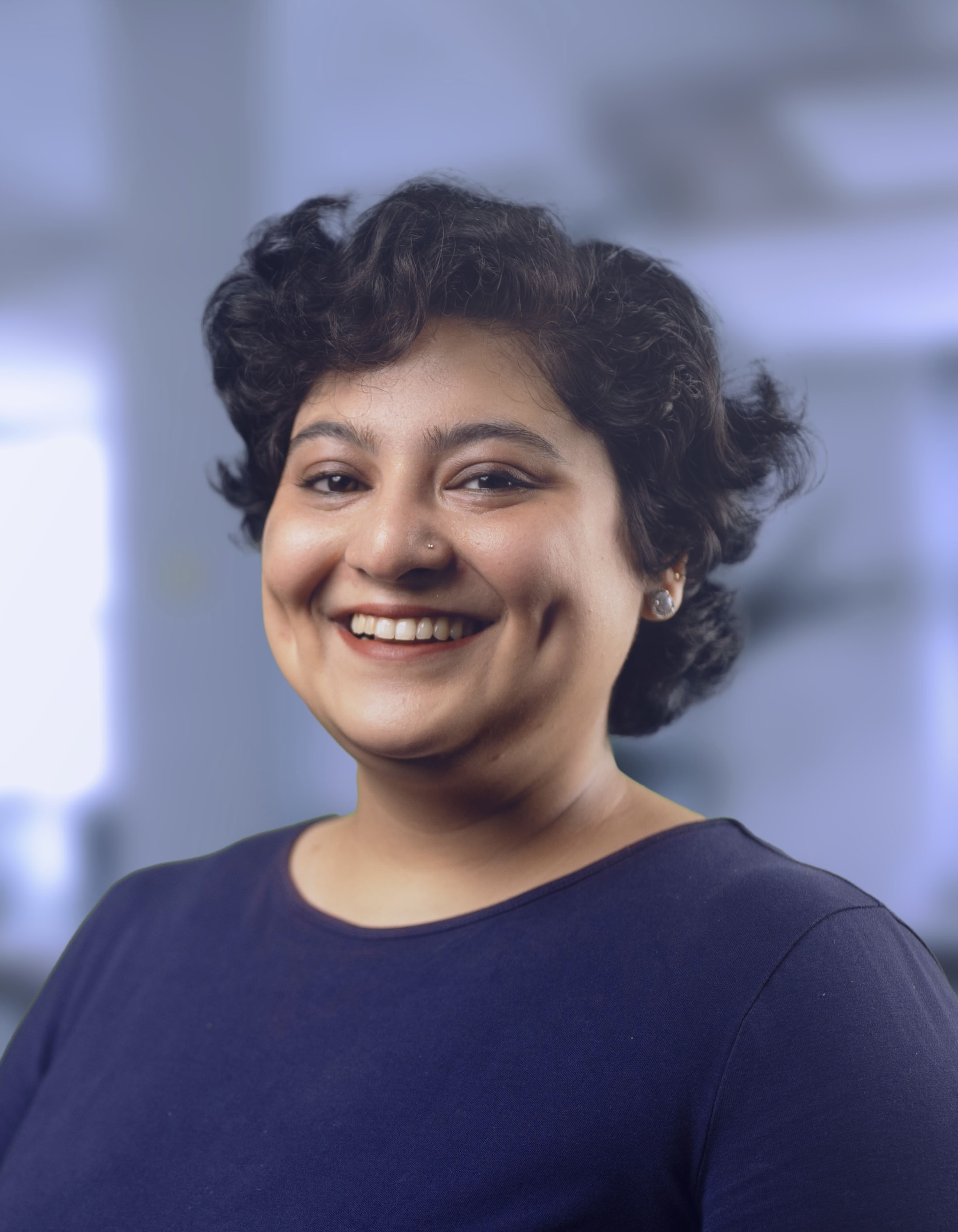
Kasturi Goswami
March 04, 2025
15 min
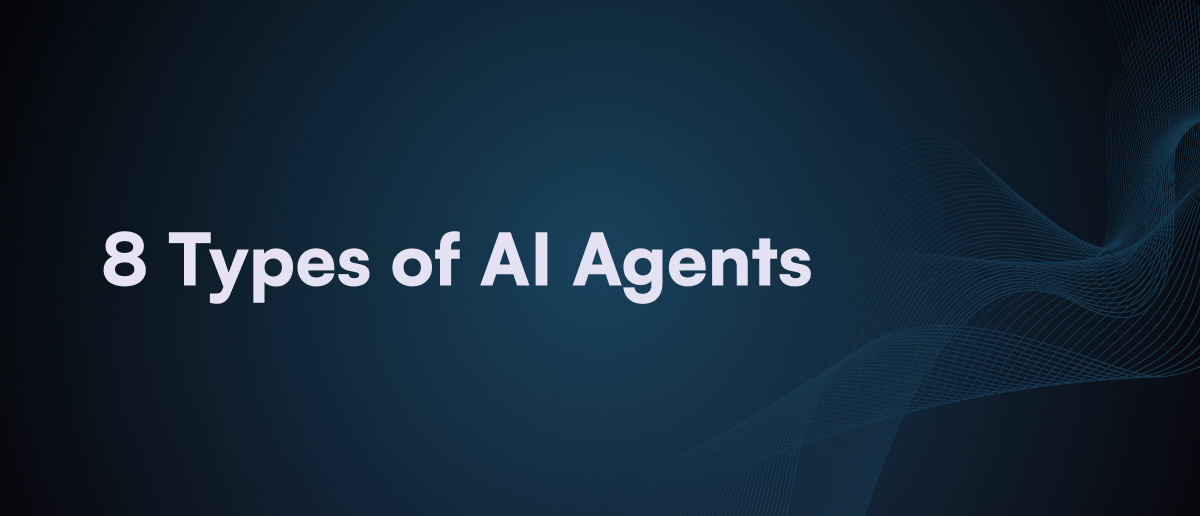
Table of Contents
Pedro had a problem.
As the CEO of a fast-growing e-commerce brand, holiday seasons brought more sales—and more chaos. Orders spiked. So did complaints, delays, and burnout. Hiring more staff didn’t scale.
To fix it, Pedro turned to AI. He implemented chatbots and rule-based automations. But instead of relief, his team got more overwhelmed. The bots were rigid, reactive, and couldn’t adapt to real-world complexity.
That’s when Pedro realized: not all AI agents are created equal.
Some follow scripts. Others learn, adapt, and make strategic decisions. The key isn’t just using AI—it’s choosing the right type. So Pedro reframed his questions:
✔ What tasks truly need automation?
✔ How much adaptability is required?
✔ Should the AI just follow rules—or evolve?
Those questions changed everything. And they can do the same for your business.
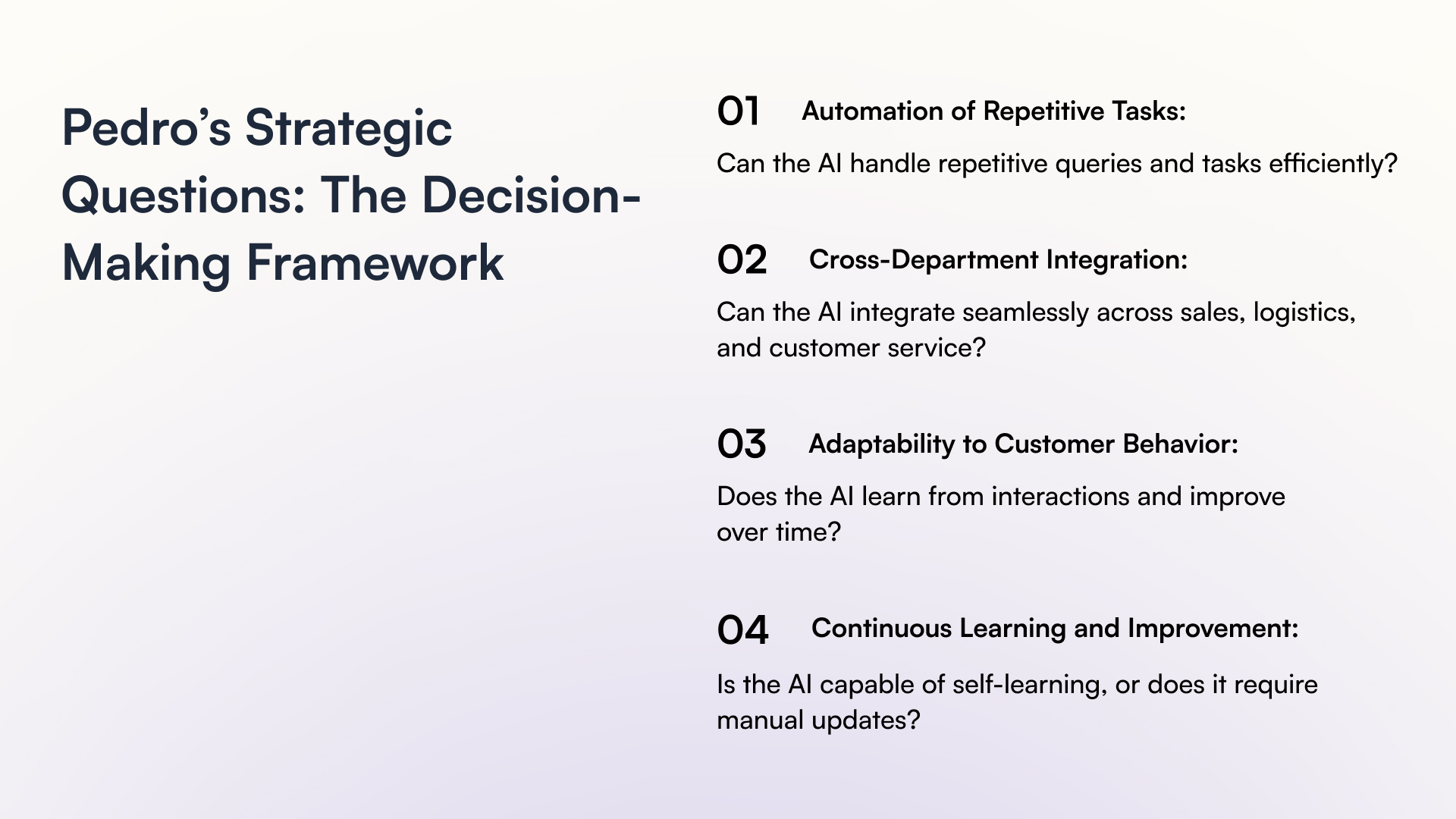
TL;DR: choosing the right AI agent
Choosing the right AI isn’t just about technology. It’s about aligning AI capabilities with business goals. The wrong one slows you down. The right one changes everything.
Here’s a quick breakdown of the 8 types of AI agents based on intelligence and fit:
Simple Reflex Agents – Fast, rule-based responses; best for basic automations.
Model-Based Reflex Agents – Use the internal state for context, but require manual updates.
Goal-Based Agents – Choose actions based on desired outcomes; limited flexibility.
Utility-Based Agents – Make optimal decisions based on measurable preferences; data-intensive.
Learning Agents – Adapt and improve over time; require training and feedback loops.
Autonomous Agents – Operate independently in real-time; ideal for dynamic, hands-off environments.
Multi-Agent Systems (MAS) – Multiple agents working together; scalable but complex to coordinate.
To scale his operations intelligently, Pedro tested several AI agent types before finding his right fit.
Are you ready to find the perfect AI agent?
Keep reading to map the best-fit AI agent to your business.
1. Simple reflex agents
The “If this, then that” automation bots
How they work
Simple reflex agents react instantly to current inputs—with no memory or historical awareness. They follow predefined condition–action rules to perform specific tasks.
- Perception: Detect input from the environment (e.g., a user message or sensor signal)
- Condition check: Match input to a pre-programmed rule
- Action: Execute the linked response instantly
Core components
- Sensors: Input sources (e.g., message detectors, motion sensors)
- Condition–action rules: The decision logic (if X-then Y rules)
- Actuators: The response/output mechanism (e.g., chatbot reply, system action)
Best suited for
- Predictable, fully observable environments
- High-volume, repetitive tasks with fixed logic
Common use cases
- Auto-responders (e.g., “reset password” bots)
- Thermostats that trigger based on thresholds
- Motion-based lighting or obstacle-avoidance robots
- Vending machines and safety sensors
2. Model-based reflex agents
Context-aware responders with memory
How they work
Model-based reflex agents build on the basic reflex model by maintaining an internal representation of the environment. They combine current inputs with past data to infer missing information and react more intelligently in dynamic or partially observable environments.
- Perception: Detect input from the environment
- Internal state update: Use prior data to update a mental model of the world
- Condition check: Match the updated model to decision rules
- Action: Perform the best-matched action
Core components
- State tracker: Maintains an internal view of the environment using past sensor data.
- World model: Encodes how the world changes and how actions affect it.
- Reasoning module: Uses the updated internal model to determine the best next action.
Best suited for
Dynamic environments with changing conditions and incomplete visibility.
Common use cases
- Advanced customer support bots that handle multi-turn conversations
- Virtual assistants that remember past commands
- Warehouse robots that navigate changing layouts
- They don’t just respond to isolated inputs.
- They take historical inputs or prior states into account.
- Their decisions reflect a broader understanding of the environment.
Are Model-Based Agents Context-Aware?
-
Yes. They are context aware, in the sense that:
By maintaining an internal model, they can infer missing information and make more informed choices—even when the environment is partially observable.
But here’s the caveat: They aren’t adaptive like learning agents.
Their internal model must be manually updated or retrained. That means while they use context, they don’t learn from it
They’re smart—but only as smart as the model they’re given.
Learn about context aware intelligence, which moulds the customer experience scene.3. Goal-based agents
AI that plans ahead to meet business objectives
How they work
Goal-based agents don’t just react—they plan. These agents compare the current state to a desired goal and make decisions based on how well actions lead toward that outcome.
- Goal initialization: Define desired result
- State evaluation: Compare current status to goal
- Planning: Sequence actions to close the gap
- Execution: Follow and adapt the plan as needed
Core components
- Goal state definition: Specifies what the agent is trying to achieve.
- Planning mechanism: Creates sequences of actions to reach the goal.
- State evaluator: Compares current state against the desired goal state.
- Action selector: Chooses actions that close the gap between current state and goal.
Best suited for
Situations that require strategic planning, multi-step execution, or navigation toward a defined outcome.
Common use cases
- Self-driving cars adjusting route based on traffic
- Smart assistants scheduling meetings around user availability
- Sales bots guiding users through lead conversion funnels
4. Utility-based agents
Decision-making AI that chooses the best possible outcome
How they work
Utility-based agents use a mathematical utility function to evaluate all possible outcomes and select the one with the highest payoff. They are like goal-based agents, but smarter: factoring in trade-offs, probabilities, and preferences.
- Define utility function: Assign value to each outcome
- Evaluate the state: Score possible actions
- Choose action: Select based on highest expected utility
- Execute & re-evaluate: Monitor results and adjust
Core components
- Utility function: Assigns numerical value to possible outcomes based on desirability.
- State evaluator: Scores different scenarios using the utility function.
- Decision engine: Chooses the action with the highest expected utility.
- Environment model: Predicts how actions will influence outcomes.
Best suited for
Decision-intensive environments like recommendations, pricing, logistics, and finance.
Common use cases
- E-commerce recommenders that personalize offers
- Financial bots optimizing trades for maximum return
- Support bots choosing the best response based on sentiment + urgency
5. Learning agents
AI that evolves and improves autonomously
How they work
Learning agents start with basic knowledge but improve through experience. Using feedback loops, they refine performance over time without human micromanagement.
- Initialize knowledge: Basic rules or models
- Observe & act: Interact with the environment
- Feedback loop: Get performance evaluations
- Learn: Update strategy based on outcomes
- Repeat: Improve with every cycle
Core components
- Performance element: Handles core decision-making and actions.
- Learning element: Improves decisions based on past performance.
- Critic: Evaluates the results of actions and provides feedback.
- Problem generator: Suggests exploratory actions to improve long-term learning.
Best suited for
Environments that evolve or require long-term optimization.
Common use cases
- Healthcare agents that improve diagnoses with more data
- AI in games that get harder based on your skill
- Customer service bots that adapt to changing language/tone
6. Autonomous agents
Self-operating AI with real-time decision-making power
How they work
Autonomous agents operate independently in real-world environments without requiring human oversight. They sense, decide, and act on their own—often in unpredictable or fast-changing conditions.
- Sense: Detect real-time inputs from the environment
- Evaluate: Analyze inputs and current state
- Act: Choose and perform the best action
- Adapt: Adjust autonomously to environment changes
Core components
- Perception system: Continuously gathers input from real-world sensors or data streams.
- Evaluation engine: Interprets input and assesses current context.
- Decision-making unit: Selects actions without human input.
- Adaptive feedback loop: Adjusts future actions based on results and environment shifts.
Best suited for
Environments that are fast-paced, decentralized, or where continuous human intervention isn’t practical.
Common use cases
- Delivery robots navigating crowded cities
- Autonomous cybersecurity systems responding to threats
- AI assistants managing entire back-office workflows
7. Multi-agent systems (MAS)
AI teamwork—agents that coordinate, communicate, and collaborate
How they work
MAS involves multiple agents—each with its own role—working together toward shared or individual goals. They gather data, communicate with one another, and take coordinated action.
- Perceive: Each agent detects inputs via its own sensors
- Communicate: Agents share insights and coordinate roles
- Decide: Each agent chooses the best move based on goals + context
- Act: Carry out actions as a coordinated unit or independently
Core components
- Individual agents: Each has its own sensors, goals, and decision logic.
- Communication protocols: Standardized methods for agents to share information.
- Coordination mechanisms: Ensure agents work together and avoid conflicts.
- Shared environment: A common space or dataset where agents operate and interact.
Best suited for
Tasks that require division of labor, real-time coordination, or geographical distribution.
Common use cases
- Swarms of delivery drones distributing packages
- Warehouse bots coordinating inventory movement
- Support agents routing customers based on language or urgency
Single-Agent Systems (in contrast)
Most AI applications today operate as single-agent systems—meaning one intelligent agent works independently within a specific environment to complete its task.
Whether it's a chatbot answering FAQs or an AI assistant scheduling meetings, the agent perceives, decides, and acts on its own, without needing to coordinate with other agents.
While simple and efficient for isolated use cases, single-agent systems can become bottlenecks in complex workflows.
8. Hybrid agents
The best of all worlds—rules, goals, learning, and flexibility
How they work
Hybrid agents combine multiple AI architectures—reflexive, model-based, goal-driven, utility-optimized, and learning—into one unified system. They are engineered to operate flexibly in diverse environments.
- Layered behavior: Mix of reactive responses and goal-driven planning
- Memory + Context: Internal models inform decisions
- Utility-based reasoning: Weighs trade-offs in outcomes
- Learning: Continuously evolves from past performance
Core components
- Layered architecture: Combines reactive, deliberative, and learning components.
- Internal state memory: Stores historical data and context.
- Utility & goal modules: Evaluates long-term objectives and trade-offs.
- Learning system: Continuously adapts across multiple logic layers.
Best suited for
Environments that need a balance of automation, adaptability, long-term learning, and context-awareness.
Common use cases
- AI copilots that assist in enterprise workflows
- Enterprise virtual assistants managing both support and analytics
- Smart manufacturing systems combining monitoring, prediction, and control
- A reflex agent handles quick responses,
- a goal-based agent manages workflows,
- and a learning agent improves everything from the top.
-
Heard about Hierarchical agents and also curious as to why we haven't included it in this list?
Hierarchical agents aren’t a separate type—they’re a design architecture. They structure how different agent types (like reflex, goal-based, or learning) work together in layers. For instance,
How to choose the right AI agent (without overcomplicating it)
The key to successful AI implementation lies in choosing the right agent for the job, not the flashiest one on paper.
Before building, start by asking:
“What is the actual job I want my AI to do?”
Once that’s clear, weigh your options against five real-world decision factors:
1. Complexity vs. task requirements
Map the task’s complexity first.
- Use simple reflex agents: if you’re automating static queries or responses.
- Use learning or hybrid agents: if your workflows change often or need memory and personalization.
Tip
-
"Avoid using a heavyweight model where a simple one will do."
2. Cost vs. capability
Complex agents require more than just a development budget—they need data, infrastructure, and oversight.
- Reflex and model-based agents are cost-effective for repetitive needs.
- Utility-based and hybrid agents shine for nuanced decisions, but they require tuning.
Tip
-
"Start with lightweight agents, then scale into complexity as ROI emerges."
3. Scalability
Some agents crumble under pressure. Others scale effortlessly.
- MAS (Multi-agent systems) scale across departments or high-volume processes.
- Goal-based agents scale well across use cases with similar structures.
Tip
-
"If you plan to grow, choose an architecture that grows with you."
4. Integration & ecosystem fit
The most advanced agent is useless if it can’t connect to your stack.
- Does it plug into your CRM?
- Can it call external APIs?
- Will it work across teams?
Tip
-
"Integration determines value—not just intelligence."
5. Monitoring & feedback
You need to improve over time.
- Learning agents excel here—if monitored carefully.
- Utility-based agents also require recalibration to stay in sync with KPIs.
Tip
-
"Whatever agent you use, make performance measurable and improvable."
6. Transparency & trust
If your business touches finance, healthcare, or customer data, ethics matter.
- Is the agent auditable?
- Can its decisions be explained?
- Is it aligned with compliance and bias detection?
Tip
-
"AI doesn’t have to be a black box—just choose agents that don’t hide their logic."
Pedro’s journey — comparing all 8 AI agent types
Pedro didn’t just pick an AI and hope for the best. He ran experiments across departments and use cases. What emerged was a real-world performance comparison, revealing which agents worked—and which fell short.
Here’s how each agent performed against Pedro’s business goals:
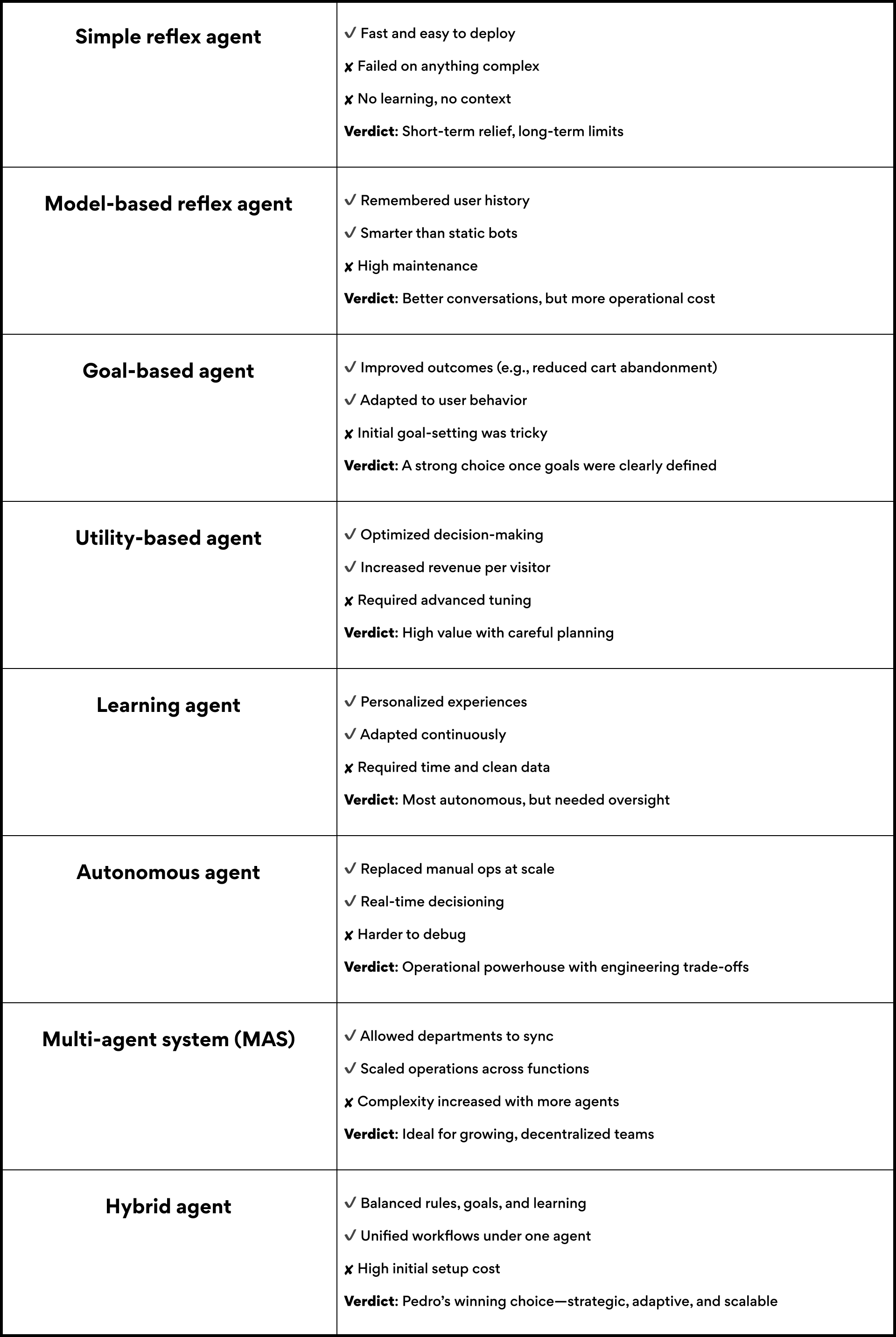
What do most businesses really need from AI agents?
Pedro’s journey wasn’t about picking the most advanced AI model.
It was about matching AI behavior to business outcomes.
He tried simple, model-based, goal-based, utility, and learning agents—but it was the hybrid combination that truly scaled with his business.
Not because it was the most technical.
But because it worked.
Now, Pedro didn’t need a textbook hybrid AI system with architected layers and complex orchestration. He needed results.
He needed a system that could respond instantly, adapt to context, learn from experience, and align with goals—all without building a separate AI team or writing a single line of code.
That’s why platforms like Thinkstack.ai matter.
-
Additional interesting reads:
The smart way to build hybrid-like AI agents without the complexity
Hybrid AI agents, by definition, combine multiple layers of intelligence—reflex logic, contextual memory, goal planning, utility evaluation, and learning.
Building that from scratch requires:
- Custom orchestration logic
- Deep AI expertise
- And weeks (if not months) of engineering time
But most businesses don’t need theoretical purity.
They need something that acts like a hybrid agent—without all the architectural complexity.
No-code agent builder platforms like Thinkstack let you replicate that same outcome—without needing a PhD or an engineering team.
Just logic that works. In workflows that matter. Thinkstack shines here:
✅ Build reflex-style automations
✅ Guide users through goal-driven flows
✅ Trigger dynamic, utility-like decisions
✅ Improve experiences using learning loops
✅ Integrate all of it—without code
Thinkstack.ai gives teams the power to simulate hybrid agent behavior, functionally—without needing to architect it formally.
It’s not about mimicking complexity. It’s about delivering intelligence where it counts.
You don’t need the smartest AI. You need the right one.
Ready to find your right-fit agent?
Your Pedro moment might be just one smart build away.
FAQs
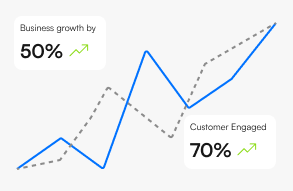